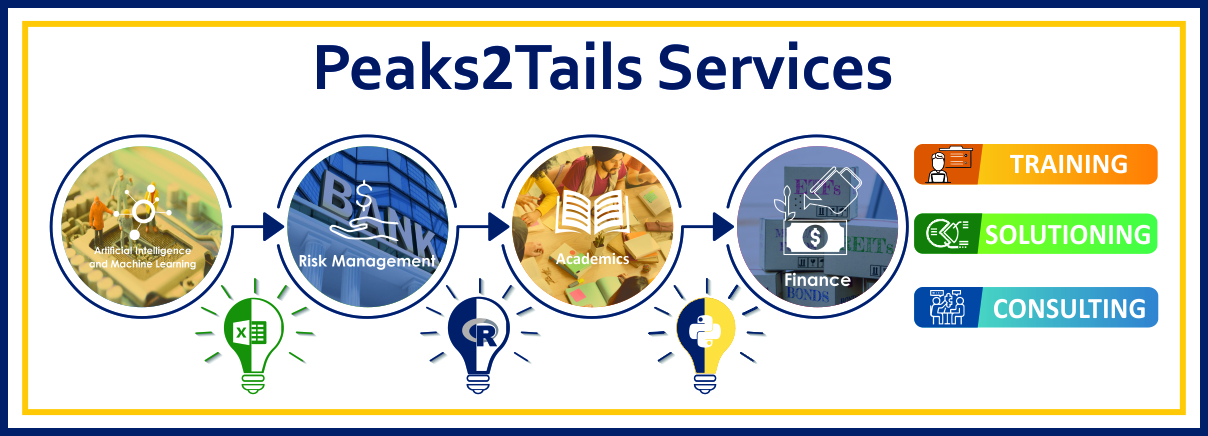
2)There should be no relationship between the residuals (error) terms. Absence of this phenomenon is called Auto Correlation. The presence of correlation in error terms reduces model’s accuracy. This usually occurs in time series model. If the error terms are correlated, it underestimates the true standard error.
3) The independent variables should not be correlated. Absence of this phenomenon is called Multi-collinearity. When predictors are correlated, the estimated regression coefficient of a correlated variable depends on the other predictor available in the model.
4) The error terms must have a constant variance. The presence of non-constant variance is called heteroscedasticity. When this phenomenon occurs, the confidence interval for out of sample prediction tends to be unrealistically wide or narrow.
5)The error terms must me normally distributed.